How do your customers feel when they engage with your brand? This is an important question to answer because customers rely on their emotions (how they feel) or can be influenced by the emotions of others when deciding on a product or service.
Figuring out what your customers feel when they engage with your brand can be the difference between business success or failure. This is where customer sentiment analysis comes in!
Sentiment analysis helps you measure customer emotions, giving actionable insight into what to offer to make your customers happy.
But what exactly is customer sentiment analysis, and how can you use it to boost your business efforts? This comprehensive guide will answer all those questions. Let’s get started.
What is Customer Sentiment Analysis?
Customer sentiment analysis is the process of examining online communication to find out how customers feel about your product or service.
Sentiment analysis involves fine-combing customer data to identify specific emotions. It helps determine whether customers have positive, neutral, or negative views of your brand.
Positive sentiments are usually expressed with words like “happy,” “good,” “wonderful,” “great,” “recommend,” etc. Words such as “bad,” “hate,” “terrible,” “sucks,” “can’t recommend,” etc. are associated with negative sentiment. Neutral reviews are conveyed with words like “fair,” “average,” “don’t know,” “maybe,” “can’t say,” etc.
Deducing the specific emotions that your customers are having when they engage with your products will give you insights that will help you make the right decisions. For example, knowing your customers’ sentiments on variables like product features can help you with product improvement. When you know aspects of your product that are frustrating customers and features they’d love to see in the product, you can tweak your product accordingly, offering exactly what makes your customers happy.
Netflix used customer sentiment analysis in this way with great results. The company’s sentiment analysis revealed that people were frustrated with certain glitches in the Netflix app, especially after installing some updates. Knowing this, the company worked on solving the technical glitch.
Also read: Customer Service Analytics Explained
Sources of Sentiment Analysis Data
The sources of reliable customer data for customer sentiment analytics include:
- Customer feedback
- Support interactions
- Social monitoring
- Customer reviews
- In-app ratings
- Voice of the customer programs (VoC)
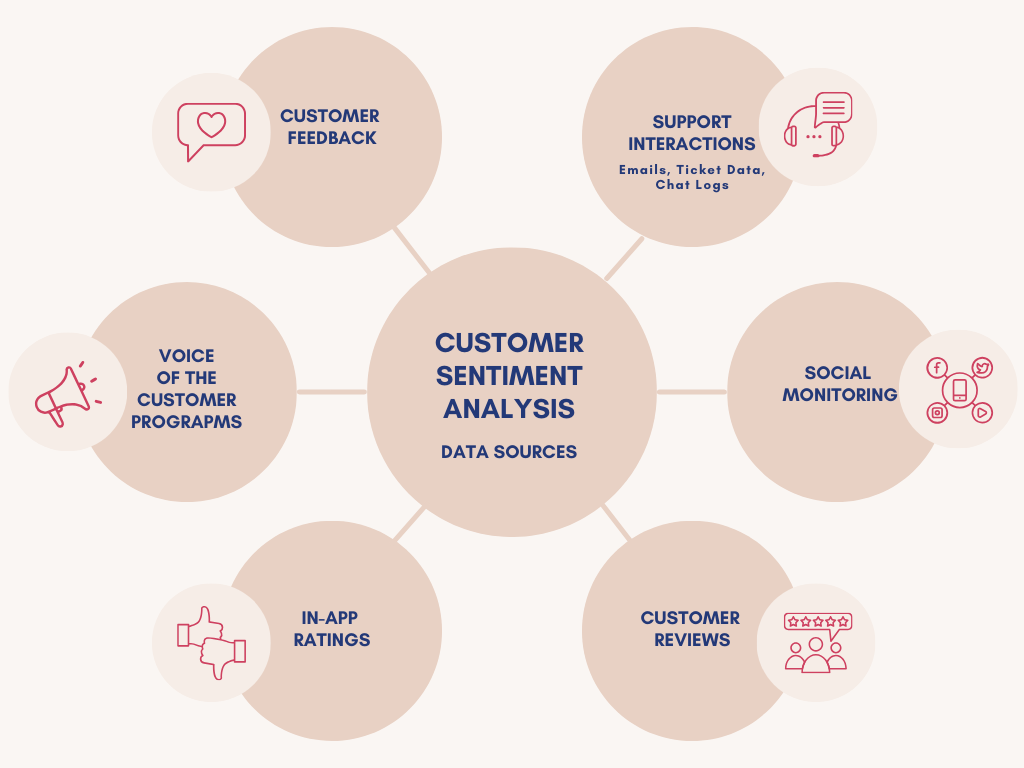
Customer Feedback
Customer feedback refers to information your customers provide about their experience with your brand. While all types of customer feedback are great for sentiment analysis, direct customer feedback is especially useful.
Direct customer feedback is obtained when you specifically reach out to customers to request their thoughts/opinions.
This type of customer feedback is one of the best for sentiment analysis because each customer can immediately see your intention for requesting their thoughts. Also, it gives them the feeling that you value their opinions.
To gain accurate information about customer sentiment, request information on as many touchpoints as possible. You can reach out to customers to collect feedback by doing the following:
- After resolving support tickets (ask them how they’ll rate their experience
- After a new feature/ product is launched (ask them what they think of the feature)
- Via website surveys
Support Interactions
Support interactions refer to any communication between customers and your customer service representatives.
Customers who reach out to you seeking help are good data sources for sentiment analysis because they tend to offer more personal information.
Customers reaching out to support usually have one issue or another, making these interactions good sources of neutral and negative sentiments.
When you look at support communication for your customers’ issues, you’ll easily see the emotions they are experiencing, giving you insights into creating a better customer experience.
Some examples of customer care interactions for sentiment analytics include:
- Support emails
- Support tickets
- Support chat logs
- Support call logs
Also read: Service Desk Basics: Cost Per Ticket
Social Monitoring
Social monitoring means tracking what your customers are saying about your products/ services and brand on social media platforms. It involves tracking data such as mentions, comments, likes, and hashtags.
About 5 billion people around the world use social media to stay connected, express themselves, and share their thoughts. These people include your customers, and their thoughts include positive and negative reviews about your products and brand (from praise to complaints and everything in between).
Thus, social monitoring is a good way to obtain reliable data to track customer sentiments. Because of the real-time nature of social media, social monitoring enables you to respond to custom complaints in real time, helping you improve customer satisfaction.
Customer Reviews
Review sites allow customers to share their opinions and ratings about specific products and brands. Some of these platforms serve specific industries (such as TripAdvisor for the tourism industry), while others are generic (like Trustpilot).
These platforms allow customers to share the experience they may have had with a product or brand, good or bad. So, they are good sources for positive and negative sentiments. Monitoring them will give you insight into aspects of your business that your customers like and areas they are unhappy with.
In-app Ratings
An in-app rating is a score that users assign to a mobile application within the app, indicating their overall satisfaction with the app.
In-app ratings usually come in the form of star ratings, ranging from one to five stars (where one represents the lowest and 5 represents the highest rating). Individual star ratings are then used to compute a summary rating for your app.
In-app ratings also come with reviews, as users are allowed to explain the reasons for their rating. These ratings and accompanying comments about the app can help you deduce customers’ emotions toward making decisions that improve customer satisfaction.
Voice of the Customer Programs (VoC)
A Voice of the Customer (VoC) program is the entire process of collating customer feedback in one place, analyzing the data to see what customers care about and why, and acting on the customer feedback to create a customer-centric culture.
Companies that effectively run Voice of the Customer (VoC) programs enjoy 10 times greater year-on-year increases in annual company revenue compared to others.
You can start a VoC program with one customer survey type, like Net Promoter Score (NPS) or Customer Satisfaction Score (CSAT). This helps you make customer-centric decisions. You collect data from traditional and non-traditional sources for analysis to understand how your customers feel at different stages of their customer lifecycle, then act on the data.
Manual Customer Sentiment Analysis
Manual customer sentiment analysis is the process of deducing customers’ emotions by manually examining customer feedback data. Manual sentiment analysis does not involve using specialized software to extract customer emotions.
The steps for performing manual sentiment analysis include the following:
Choose Feedback Channels
Decide on the channels you want to collect custom feedback from for sentiment analysis. For effective analysis, it’s best to collect data from different sources, especially data from support interactions, customer reviews, and social monitoring.
Collect Customer Feedback
Next, collect the customer feedback data in one place. Google Sheets and Excel are popular for manual sentiment analysis for support.
Categorize the Feedback
Read the text of each feedback, then use the language, tone, and context to separate it into positive, negative, or neutral sentiments. Certain words can immediately reveal that feedback reveals a positive or negative customer sentiment.
For example, “happy” in the feedback, “I am happy with the product,” shows positive sentiment.
But sometimes, language alone may be misleading (hence the need to use tone and context when categorizing feedback). For example, while “long time” may reveal a positive sentiment for product durability, it reveals a negative sentiment for customer service time.
Identify the Topic Each Sentiment is About
Each category of feedback will cover different topics. To help you understand what your customers are happy or unhappy about, you should dig deep into each feedback to identify the topic each sentiment is about.
Thus, you can link customer’s emotions to specific aspects of your business (e.g., product availability, ease of use, customer service wait time, etc.).
Rank the Sentiments
Customer emotions are not equal, so it is best to use the tone of each feedback to determine the severity of the expressed emotions.
Consider the two negative feedbacks below:
- I am not happy with XYZ’s extra-slim model.
- The XYZ’s extra-slim model sucks. It’s an absolute disgrace that such crap can come from a company of XYZ’s standing.
While both feedbacks show negative sentiments, it’s clear that the second is more damning than the first. So, having them on an equal footing will not reflect people’s real feelings appropriately.
This is where using sentiment scores comes in. A sentiment score lets you rank each sentiment, helping you consider the weight/ severity of customers’ sentiments during analysis.
Analyze the Recorded Sentiments
After identifying and ranking customer sentiments, proceed to analyze them to understand patterns and trends in customer feedback.
For example, you can look at the most common themes (to identify aspects of your business that elicit the most customer engagement), the themes with the highest sentiment score (to identify what your customers are least pleased with), etc.
This analysis offers eye-popping insights about your business, such as identifying areas for improvement.
Challenges of Manual Sentiment Analysis
Extracting customer sentiment manually is only possible if you’re a small organization and your customer feedback data is not very large.
Even when you “fit the bill,” going at it manually comes with challenges, such as:
Stressful
Manual sentiment analysis involves a lot of work, as it involves manually looking for customer feedback, importing them to a spreadsheet, reading and sorting them, etc. This is what makes manual sentiment analysis impractical when you have a large volume of data.
Time-consuming
The process of manually tracking user sentiment is a long one. The time spent can be used for more productive activities.
Bias
Manual sentiment analysis can be full of human biases. For example, bias enters when assigning scores to sentiments to establish their weight/severity.
Automated Customer Sentiment Analysis Tools
Automated customer sentiment analysis uses specialized software to deduce the emotions that people feel when they engage with your products or brand.
Automated customer sentiment analytics tools help you avoid the challenges of manual analysis. Some of the benefits include:
- The tools take the work off your hands so you gain insights without stressing yourself.
- They give you more accurate insights as the automated tools eliminate human error and biases.
- They serve you valuable insight quickly as they can crunch large volumes of customer feedback data quickly.
These tools are broadly divided into two categories:
Lexicon-based software
These tools know a vocabulary of words/ terms, which they associate with the different emotions (positive, negative, and neutral). They scan customer feedback data for these keywords and use them to calculate the overall sentiment of the feedback.
However, these tools struggle to understand linguistic nuances (like sarcasm, irony, etc.) and ambiguities.
For example, consider the feedback, “Thank God this item finally arrived.” A lexicon-based sentiment analysis tool will not see the sarcasm in the feedback and will classify it as positive.
AI-based software
These tools use machine learning and natural language processing (NLP) to provide more accurate sentiment analysis. Natural Language Processing allows AI-based tools to easily solve many of the problems of lexicon-based tools.
The algorithms help the tool to look beyond keywords but also analyze context. So, it can detect sarcasm, irony, negation, etc.
Some of the top automated tools for analyzing customer sentiments include:
MonkeyLearn
MonkeyLearn is a powerful AI platform that lets you use machine learning to extract text from various sources (such as email, chats, and documents) and analyze them for insight, saving you hours of manual data processing.
MonkeyLearn is easy to use. You simply connect to your data, quickly turn your text into tags using premade models, and use the tags to extract new information about your business.
The software integrates with over 1,000 web tools (including Zendesk) and is extremely easy to use.
Also read: The Benefits of Text Analysis For Support Teams
Rosette
Rosette is an AI text analytics solution that understands human languages. Language identification is the first step in any text analysis or natural language processing (NLP) pipeline.
If the tool misunderstands the language, all subsequent models will produce inaccurate results. This is where Rosette Text Analytics shines.
When customer feedback data has multilingual text, Rosette is a great tool for accurately analyzing it for customer sentiment.
The software can extract key pieces of information from unstructured data and interpret content in a fraction of the time it takes many other applications.
Rosette can be easily integrated with applications you already use via APIs, allowing optimization without costly system replacement.
Clarabridge
Clarabridge is an AI-powered text and speech analytical tool that can help you collect and analyze customer feedback across several touchpoints.
Its natural language processing engines evaluate text to determine contextual meaning, helping you deduce customer emotions accurately.
It uses built-in categorization, an 11-point sentiment scale, semantic analysis, and more.
It also integrates with your existing CRMS, allowing you to analyze customer sentiments comprehensively.
Idiomatic
Idiomatic advertises as an AI-driven customer intelligence platform. The platform can help you track customer sentiment and identify the “why” behind your customer feedback.
You can create custom data labels organized in easy-to-understand categories, surfacing trends you may not have seen before. The tool offers different sentiment analysis models for each data source. This helps you track more accurate sentiments by channel.
Getting Started with Customer Sentiment Analysis
Below is a step-by-step guide for beginners to start tracking customer sentiment effectively:
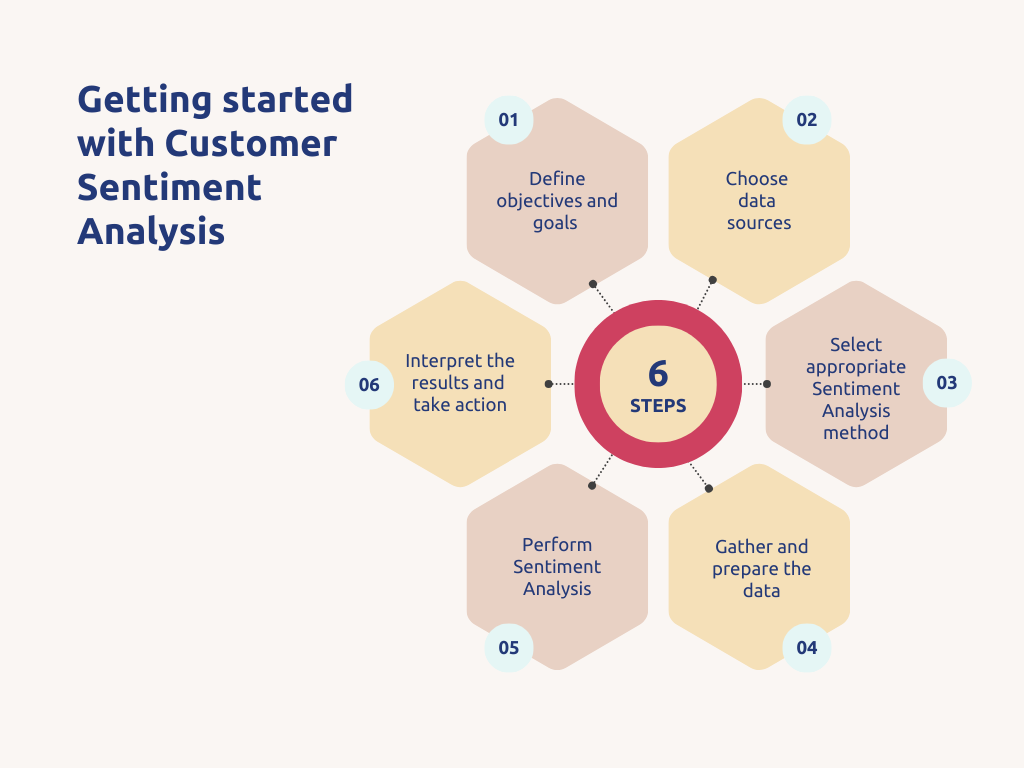
Define Objectives and Goals
Why do you want to measure customer sentiment? Answering this question helps you define your objectives and goals, giving you direction.
For example, your objectives determine the kind of feedback data you’ll use or the kind of analysis you’ll run on the data.
Choose the Data Sources that Align with your Objectives
The best customer feedback data for sentiment tracking depends on your objectives. So, after defining your objectives, the next step is to gather feedback data from the appropriate source.
For example, in-app ratings and reviews are good sources of feedback data to evaluate the sentiments of your app’s users, while social monitoring is a good data source to gauge the general public’s sentiments.
Select the Appropriate Sentiment Analysis Method (Manual or Automated)
After choosing data sources that align with your goals, the next step is to decide on an appropriate sentiment analysis method.
You may choose to manually analyze customer sentiment if your data is not large. But when you have large volumes of data, then use automated tools.
Even a small company can have 100k+ mentions in a month. It’ll be back-breaking to read through each one to deduce the sentiment.
Gather and prepare your data
You can manually upload your data to the analytic tool by downloading the user comments and feedback in a .csv file. Automated tools make things easier as you can connect to your data sources via direct integrations or APIs.
Once the data is in the pipeline, natural language processing, semantic classification, and other models clean and categorize it so that it can be analyzed for sentiments.
Perform Sentiment Analysis
After categorizing feedback and identifying themes/ aspects, perform an analysis to identify patterns in the data, determine the proportion of positive to negative sentiment, etc.
Interpret the Results and Take Action
The objective of sentiment analysis is to understand the motivation for customer behavior and take action to improve customer satisfaction.
Thus, the final step in customer service sentiment analysis is to interpret the results as they affect your business and take action.
Real-world Success Stories and Examples of Customer Sentiment Analysis
Many companies have achieved significant improvements using customer sentiment analysis. Let’s explore some of these stories and the results.
Airbnb
Airbnb is a platform that matches people looking for accommodation in a particular city to people willing to rent out their place. The company used sentiment analysis to understand the true feelings of its users.
Challenge: Airbnb guests and hosts have real-life interactions that often force the guests to leave inflated reviews for the hosts. Thus, guests’ star ratings and reviews on Airbnb were not the true feelings of guests.
Solution: Airbnb used sentiment analysis to analyze its guests’ feedback on third-party review platforms.
Result: The analysis helped Airbnb understand the true feelings of guests.
Starbucks
Starbucks, the premier retailer of specialty coffee, is another company at the forefront of using customer sentiment analysis. Starbucks uses sentiment analysis to track poor customer experience for effective management.
Challenge: Starbucks gets a lot of mentions, as there’s an average of 10 tweets about the company every second. To effectively respond to poor experiences, it needs a system to track the numerous user reviews and identify negative sentiments.
Solution: Starbucks used sentiment analysis to interpret the deluge of customer feedback it gets from social media.
Result: With sentiment analysis, Starbucks easily crunches its vast customer feedback data and compiles them to identify poor customer experiences.
Management also sifts through information to identify public opinion on different aspects of business, such as cleanliness standards at specific Starbucks locations. This helps Starbucks to respond to poor experiences quickly. It also helps the company make important decisions toward service improvement.
Netflix
Netflix is one of the biggest online streaming providers of video-on-demand distribution. The company uses sentiment analysis in different ways, including to predict the performance of certain Netflix Originals.
Challenge: When Netflix adds a movie, the marketing department will want to know what users feel about it and use this information to predict its performance.
Solution: Netflix used sentiment analysis to analyze users’ feelings about its Original series, House of Cards. The analysis looked at users’ data from Twitter and compared it with reviews on IMDb (Internet Movie Database).
Result: Sentiment analysis revealed that users highly rated the movie, and this information was used to predict that the movie would continue to lead the trend.
Benefits of Customer Sentiment Analysis
Reduces Escalations
Early detection of negative sentiments in customer communications can enable you to prompty address the issue before it escalates.
Reduces Resolution Times
Sentiment analysis helps you understand the underlying emotions in customer feedback, reducing the need for multiple back-and-forths to clarify and resolve issues.
Automated tools can also identify negative sentiments in real time and automatically route tickets to appropriate agents, allowing for increased first contact resolution or faster overall resolution.
Enhances Support Productivity
Sentiment analysis automatically classifies customer feedback based on sentiments and reveals the underlying emotions in complaints. This helps your customer service team boost productivity and ultimately offer better support.
Personalizes Customer Interactions
By understanding when a customer is happy, sad, frustrated, or angry, support agents can tailor their response accordingly and personalize the service to the needs and concerns of individual customers.
Decompresses Backlog
Since sentiment analysis can help reduce resolution time. You can, therefore, handle more support complaints, decompressing backlogs.
Anticipate and Reduce Churn
If customers have poor experiences when they engage with your product or service, they’re likelier to leave you, increasing your churn rate.
Sentiment analysis helps you understand customers’ feelings, which you can use to predict churn rates. You can then do something about it by responding to unhappy customers and doing something to fix what makes them unhappy.
Boost Customer Satisfaction, Retention, and Royalty
Sentiment analysis helps you understand what makes customers happy so you can offer more of it or what makes them unhappy so you can fix it and make them happy.
Therefore, sentiment analysis boosts customer satisfaction, which improves customer retention and brand loyalty.
Improve Products and Service Offerings
Understanding how customers feel about your products helps you identify problem areas in your offerings. You can then work to fix these, thereby improving your products and better meeting clients’ needs.
Design Better Coaching and Training Programs
Analyzing customer sentiments over time can help you identify areas where agents need improvement. This can help you create training and coaching programs to address specific agent weaknesses.
You may also integrate sentiment analysis into KPIs for agents, whereby you evaluate them based on their ability to maintain positive customer sentiment.
Common Challenges with Customer Sentiment Analysis
Ambiguous Words
Sentiment analytic tools often struggle to capture nuances of language, especially with words having different meanings.
For example, “House of Dragons is killing it.” Models will classify the sentence as negative because of the keyword “killing,” but it is positive feedback that means that the show is extremely good.
Sarcasm and Irony
People often use sarcasm and irony when expressing negative feelings. This entails using a positive-sounding word to express a negative emotion.
For example, “Thank God, the Jordans finally arrived.” Models may classify the feedback as positive when it is negative.
Double Negation
There are certain keywords that sentiment analysis models see as negative sentiments. However, negations reverse their meaning.
For example, “The pasta is not bad” or “The movie is not unpleasant.” Algorithms will classify the feedback as negative because of the keywords “bad” and “unpleasant.” However, the negations before these words make the sentences positive.
Subjectivity
Sentiment is subjective and can vary from person to person. What one person considers as negative may be neutral or positive to another. Sentiment analysis models do not account for these differences.
Best Practices and Tips to Make the Most of Sentiment Analysis
The following will improve the accuracy of sentiment analysis results:
High Quality Training Data
Train your algorithm with large data sets representing the domain and language you’re analyzing.
Data Preprocessing
Clean your data to remove noise (such as special characters and irrelevant information) and fix abbreviations.
Select the Right Model
Choose the sentiment analysis model that aligns with your needs. You may choose a lexicon-based or an AI-based model. Consider using a model that supports multiple languages if your data involves multiple languages.
Regular Model Update
Keep your sentiment analysis model up-to-date by periodically retraining the algorithm on new data to adapt to changing language patterns and slang.
Takeaway: Tap into Customer Sentiment to Deliver Positive Experiences
Customer sentiment analysis helps you know how your customers feel when they engage with your business.
Understanding whether your customers have positive or negative sentiments can be the difference between business success and failure.
When you identify positive sentiment, you can offer more of what generated it to improve customer delight. Additionally, when you identify negative sentiment, you can respond appropriately to solve the issues and fix the problem areas.
Thus, if you haven’t started, you should start paying attention to how your customers feel.
It’s also important to stay updated with the latest trends in sentiment analysis. Algorithms and models are continuously developed to enhance the accuracy of sentiment analysis.
This gives you a competitive advantage, as you’ll be able to understand customers more effectively, make data-driven decisions, and promptly respond to market changes.
Did you like the post?
You might also like:
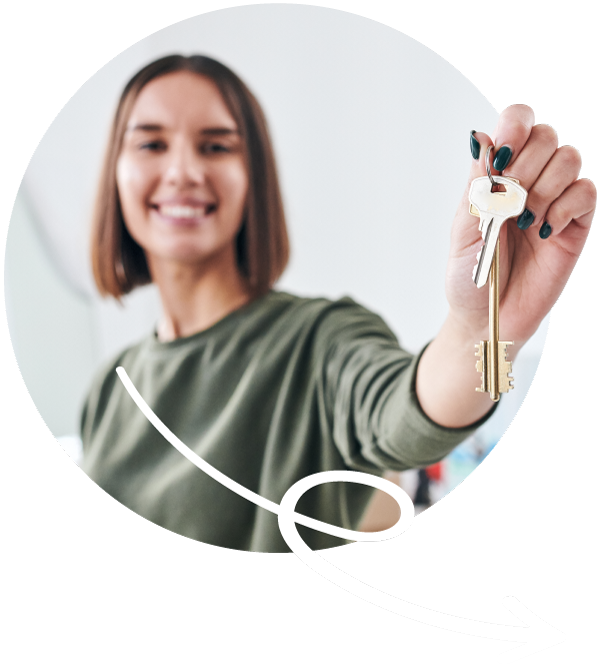
Surveypal
Everything you need to lead and improve your customer experience. Learn more at surveypal.com, or