Customer support is integral to business success. Customers expect elevated experiences when interacting with a brand, so they readily do business and even recommend brands that meet their expectations.
Text analysis is one technique helping brands meet customers’ expectations in today’s business environment. It allows you to listen to everyone wherever they are, giving you critical feedback to act on to improve the customer experience and the overall quality of your customer service.
How do you implement text analysis for customer support?
What is Text Analysis?
Text Analysis is a machine-learning technique that automatically extracts actionable insights from unstructured text. It is about using computer systems to digest online data and documents to extract meaningful information you can respond to and act on.
Text Analysis helps you to efficiently process multiple text-based sources (like documents, product reviews, service desk tickets, emails, social media content, etc.) as a human would.
Gone are the days when customers were giving feedback only via surveys.
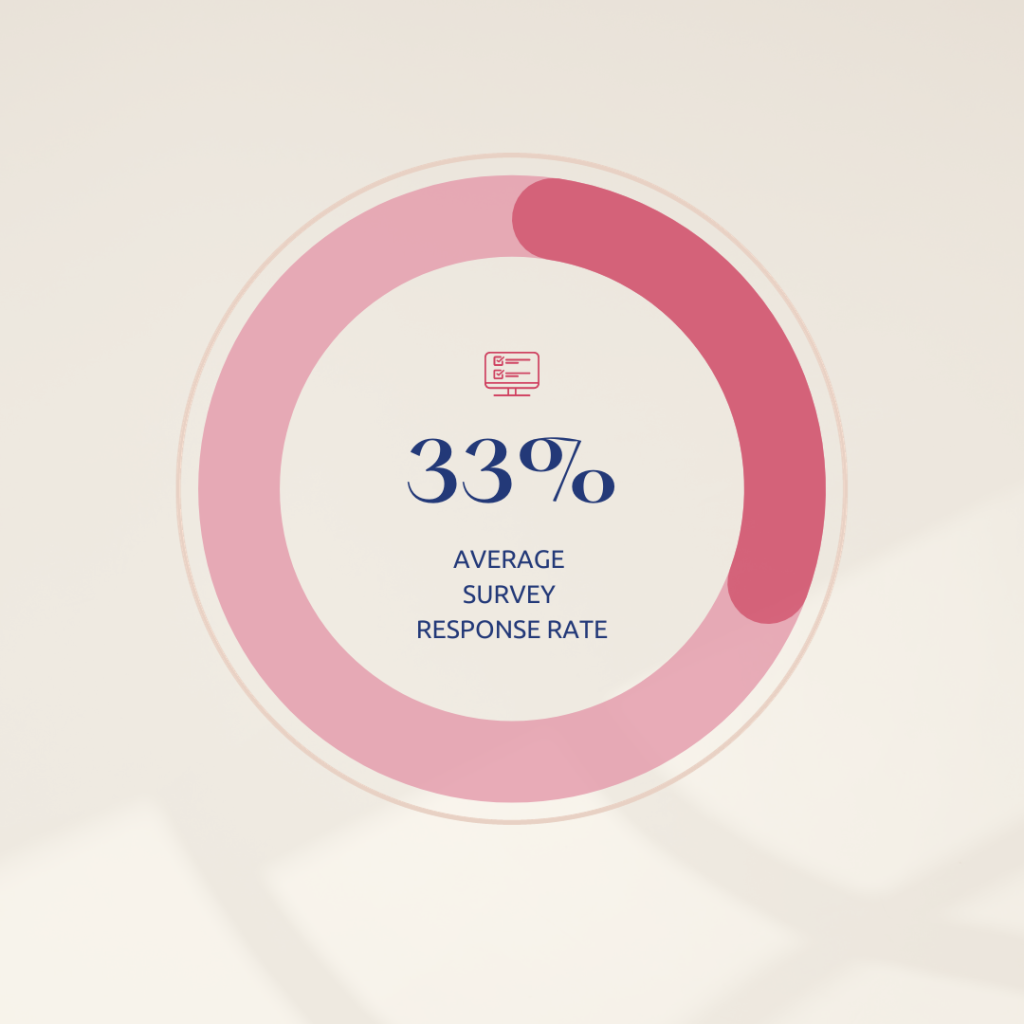
In today’s business environment, customers will reach you via email or other approved channels and discuss you on review platforms and social media.
Being everywhere simultaneously to listen to what your customers may have to say and take on issues that may come up is next to impossible.
But with Text Analysis, you can go through millions of text sources, using specific keywords such as names or company information to identify when a discussion should concern you. For example, you can monitor brand mentions on social media and get real-time alerts when anyone mentions your brand.
You can, also, automatically sort and classify the information extracted from text data to identify sentiments, patterns, relationships, and other actionable insights. Or, categorize customer sentiment into positive or negative and further separate issues into specific topics, such as order issues, service issues, etc.
Thus, Text Analysis helps you turn unstructured data into structured data, so you’ll know what your customers need.
The different types of text analysis techniques
There are different types of text analysis techniques, as follows:
Sentiment Analysis
Sentiment Analysis is about understanding the emotions your customers express about your brand or product/service experience.
Sentiment analysis uses Natural Language Processing to analyze customer sentiments from customer interactions across a variety of sources.
In its simplest forms, it tracks brand mentions, extracts customer sentiment, classifies the sentiment (as positive, negative, or neutral), and scores the sentiment to show you the real value of customers’ emotions (“the product is very good” will have a higher score than “the product is good”).
Tracking your customers’ emotions helps you make data-driven decisions to improve customer experiences and maintain brand loyalty.
Topic Modeling
Topic modeling is the process of identifying related keywords in pieces of information extracted from textual sources and grouping them into topics or themes.
Topic modeling sorts the unstructured, heterogeneous data into themes. For example, a topic modeling algorithm analyzes specific mentions to identify whether the customer’s needs fall under product quality, pricing, orders, service, etc.
Text Classification
Text classification is the process of assigning tags to text data based on pre-defined categories. The text classification algorithm relies on a set of tags to structure and summarize unstructured data extracted from the web.
For instance, if we have the statement, “I am happy with my XYZ hoodie. The quality of the material is so good,” the text classifier will take it as input, analyze it, and then use relevant tags such as “happy,” “product quality,” and “good” to summarize it.
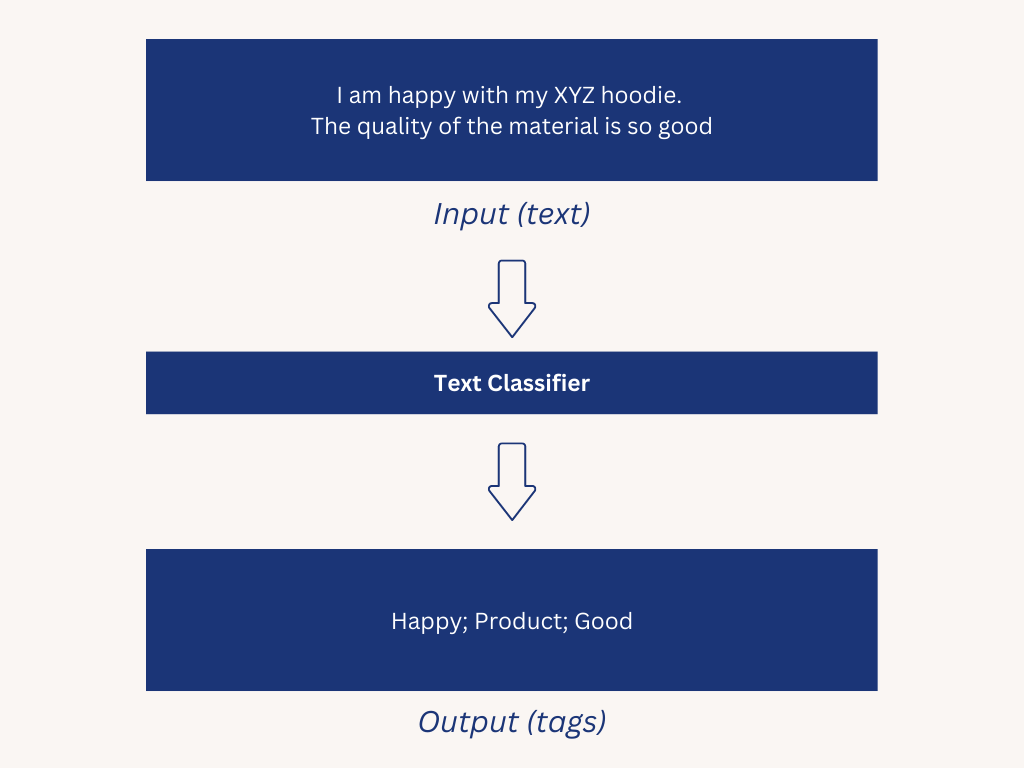
By reducing the extracted information into relevant tags, text classification makes it easy to classify them into topics, sentiments, etc.
Text Clustering
Text clustering groups textual data so that similar texts are put together.
Through this process, the algorithm analyzes data and determines whether similar objects exist in the group. If if does detect similar objects, it will separate them into clusters.
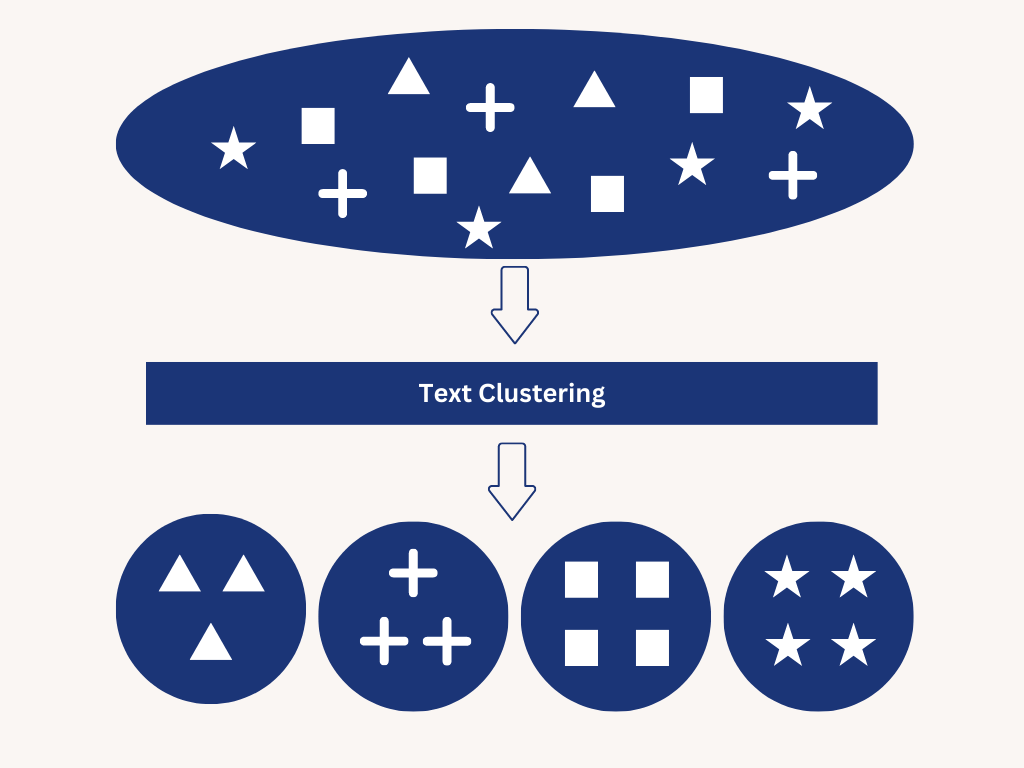
Named Entity Recognition
Named Entity Recognition (NER) is an NLP method that involves identifying and extracting specific information from unstructured textual sources.
Named entities are key subjects of textual data (such as company name, product name, locations, etc.) that concern your brand.
NER delivers key information that can help you improve customer experiences. For example, your support service can use it to detect when a customer leaves a review on a platform or mentions you on social media.
Text Analysis starts with named entity recognition. You must have a piece of information before you can analyze it to extract business insights. Named Entity Recognition scours the web and brings you information that concerns your brand.
Benefits of Using Text Analysis for Customer Service
Using Text Analysis for customer service benefits your brand in many ways, such as:
- Improved response times
- More accurate issue resolution
- Enhanced customer satisfaction
- Identifying customer sentiment
- Targetted responses to customer queries
- Pinpointing trends and pain points
- Driving product and service development
In the following paragraphs, we’ll dive a bit deeper into those benefits.
Improved Response Times
Text Analysis alerts you of customer comments/ complaints on different platforms in real time.
Thus, you can swiftly respond to customer issues and offer immediate solutions, reducing your average handle time.
More Accurate Issue Resolution
Text Analysis uses different algorithms to classify extracted information by topics and customer sentiments accurately.
This helps you streamline the process and offer more accurate issue resolution. For example, classification helps your support agents understand the customer’s needs, while sentiment analysis helps them measure customers’ true emotions.
Enhanced Customer Satisfaction
Customer satisfaction depends on meeting customer expectations, and text analysis helps you meet or exceed customer expectations.
For example, 12% of Americans rate “lack of speed” as their number one service frustration. Text analysis tracks textual data for customer complaints in real-time, allowing you to offer immediate solutions and have happy customers.
Identifying Customer Sentiment
Text analysis helps you determine how customers feel about the products and services you offer them and the experiences they receive from your brand.
Effective text analysis does not just classify customer sentiments into negative, neutral, and positive. But it scores each sentiment, helping you understand the strength of the customer’s emotions and respond appropriately.
For example, consider two pieces of customer feedback, “I am not happy with the ABC model” and “I am so angry that you guys shipped me a piece of shit as the new ABC model.”
While they are both negative sentiments, the “piece of shit” comment shows an angrier customer (and will have a weightier score).
Thus, sentiment analysis helps your agents know the type of customers they’re dealing with, how to prioritize tickets, etc.
Developing Targeted Responses
Text Analysis helps you to structure qualitative data, pointing you to the real issue in customer complaints, giving you a glimpse of the type of customer, etc. This helps you provide tailored responses addressing customers’ concerns and emotions.
Identifying Trends and Pain Points
When you track customers’ interactions on review platforms and social media, you can spot common pain points and issues before they begin trending.
Text Analysis dives deep into information extracted from textual sources and analyzes the said and the unsaid to understand what a customer really wants or expects. This can also help you predict customer behavior and be ready to meet them, increasing customer satisfaction.
Drive Product and Service Development
The first step to solving a problem is identifying it.
Understanding how your customers feel about your product and service helps you take steps to improve your offerings.
For example, consider that your analysis shows dissatisfaction with an aspect of your product’s quality. Then you know that to provide an excellent customer experience, you must enhance or add features to improve that quality aspect.
How to Implement Text Analysis: a Step-by-Step Guide
Many text analysis platforms have easy-to-use interfaces, allowing you to run your data and visualize results without adding a single line of code.
The simple steps for using text analysis software include:
- Choose a template. Text analytics tools offer different templates for running analysis. The first step is to choose an appropriate template.
- Upload your data. The next step is to upload your data. Tools generally accept a CSV file.
- Match your data to the right fields.
- Name your workflow: Set a name for your workflow.
- Run your data. The tool automatically processes your data.
- Visualize your data. The tool presents your analytics result using easy-to-understand dashboards. You can then visualize the result to spot trends, patterns, and other actionable insights.
How to Implement Text Analytics for Customer Support
No matter the type or size of your business, you can implement text analytics to improve customer service productivity. Implementing text analytics for customer support requires the following:
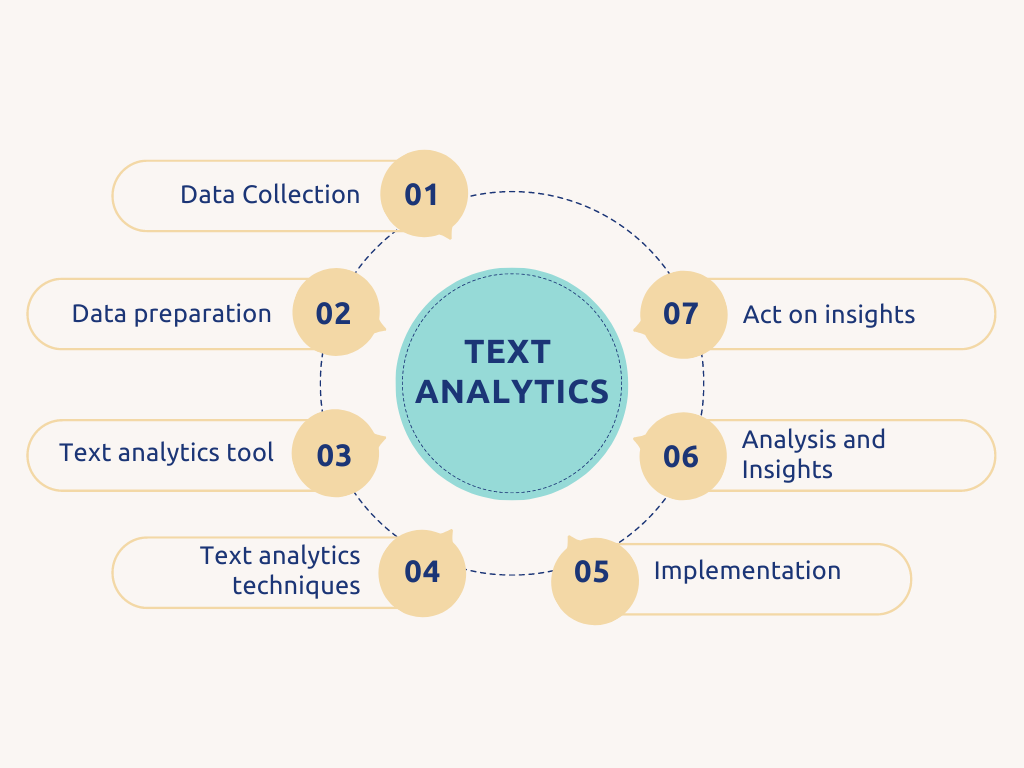
Data Collection
The first step is to collect customer support data, including chat or chat transcripts, email communications, social media interactions, and customer feedback surveys.
Collecting a significant amount of data is important to ensure accurate analysis. So, gather data from internal and external sources.
- Internal data are those internal to the business that you generate every day. Sources include emails, chats, online support tickets, etc. You can export it from your platform as a CSV or Excel file or connect to an API to retrieve it directly.
- External data is data about your brand all over from all over the web. Sources include news articles, online reviews, online forums, and social media posts. You may need web scraping tools to collect external data. Many of these platforms have their own APIs that you can use to search their archive and collect users’ comments.
Data Preparation
Once the data has been collected, it needs to be prepared for analysis. This involves cleaning the data, removing irrelevant information, and ensuring it is in an easily analyzable format.
Data preparation happens via several text analytics techniques, which include the following:
- Tokenization. Breaking the raw text into semantically meaningful parts. For example, the text “Their gadgets are good” is tokenized into: “their,” “gadgets,” “are,” “good.”
- Part-of-speech tagging. Attach grammatical tags to the tokenized text. For example, the PoS tags for the tokens above are, Their: Pronoun, Gadgets: Noun, Are: Verb, Good: Adjective.
- Parsing. Establish a meaningful connection between the tokenized words (using the grammar of the language the text is written in) to help the text analysis software visualize the relationship between words.
- Lemmatization. Simplify words into their dictionary form by removing affixes (suffixes, prefixes, etc.).
- Stop-word removal. Remove words with little or no semantic context (such as a, and, for, etc.).
However, your text analytics tool handles most of the data preparation automatically, and you won’t even notice it happening.
Text Analytics Tools
Next, you should choose a text analytics tool that can analyze customer support data.
Any decent tool should allow the detection of named entities from text, grouping content into themes, and extracting meaning from text.
Good tools should offer pre-trained text analysis models and also allow you to create custom models for analyzing text and extracting valuable insights.
Text Analytics Techniques
Always choose the text analytics techniques best suited to your business needs.
As mentioned earlier, these techniques may include sentiment analysis, topic modeling, named entity recognition, text classification, and text clustering.
Implementation
After selecting the appropriate text analytics tools and techniques, the fun part begins – the analysis process.
This involves running the data through the text analytics tool and applying the selected techniques to gain insights into service desk interactions, open-ended customer feedback, brand mentions, reviews, etc.
This process will enable you to categorize keywords and assign sentiments.
Analysis and Insights
Once the analysis is complete, you can begin to analyze the results and gain insights from your customer support conversations. This can involve identifying trends, common issues, and customer sentiment.
Visualization tools can help you turn text analysis results into easier-to-understand formats. They present analytics results in striking dashboards (graphs, charts, and other visual elements), making it easier to understand your results.
Act on Insights
Insights gained from text analytics can help extract meaning from customer intractions and make informed decisions to improve customer support. But any success depends on taking actionable steps.
For example, if analytics show that customers complain of slow response time, you should implement strategies to improve response times.
Other insight-driven actions may include addressing common issues, updated the content of your knowledge base, and developing targeted responses.
Challenges of Using Text Analytics for Customer Support
Using Text Analytics for customer support comes with challenges relating to data quality, data complexity, interoperability, and data privacy.
Data quality
Text data can be incomplete, ambiguous, and full of noise. That is, the data often contain spelling errors, abbreviations, slang, and ambiguous words, so understanding the data can be challenging.
For example, consider the customer remark, “imo, ur delivery time of 2 weeks does not make sense”
The Text Analytics tool will have difficulty understanding that
- imo = in my opinion
- ur = your
- does not make sense = too long.
Data cleaning and preprocessing helps you overcome data quality issues to successfully implement text analytics by removing noise, errors, and irrelevant information.
It also helps clear ambiguity and standardizes extracted data. Some data-cleaning techniques for making raw data easier to understand include tokenization, lemmatization/stemming, stop-word removal, and encoding.
Data Complexity
Customer feedback/complaints and other data that will interest your support teams may reside in source systems not created with analysis in mind, making extracting the data or textual analysis challenging.
For example, unstructured data can come in types or formats such as video, audio, and images. Extracting such data for textual analysis can burden the performance of the source system and take a long time. Integrating and combining textual data with other data types can also be difficult.
Data transformation and representation helps overcome data complexity issues, making it easier to combine different data types for analysis.
You can do data transformation using vectorization, embedding, and dimensionality reduction.
Interoperability
Your text analytics tool becomes part of your data infrastructure, so having software that easily integrates with your existing systems makes for seamless data consumption.
A text analytics platform incompatible with your data infrastructure can make data transfer challenging, whether transferring raw data to the tool for processing or transferring analytics results to your data system to drive action.
Overcoming interoperability issues requires choosing a text analytics tool that works well with your data infrastructure.
Privacy Concerns
Some privacy laws prohibit the disclosure of an individual’s private records without their consent. Since unstructured data may contain personally identifiable information, you risk breaching privacy laws when you do text analysis and index documents in search solutions.
Overcoming privacy concerns
PII redaction is a technique that can help you overcome privacy challenges and implement text analysis successfully without breaching privacy laws.
PII redaction automatically detects and removes personally identifiable information (such as names, contact details, addresses, etc.) from your data. Thus, the individual’s privacy is protected even when you index documents in search solutions.
Wrapping up
Text analytics enables you to dive deep into your data to identify the root cause of your performance issues and find solutions that prevent them from escalating and impacting customer satisfaction.
Using Text Analytics helps your business in many ways, including improving response time, developing targeted responses, providing more accurate issue resolution, identifying customer sentiments, enhancing customer satisfaction, identifying trends, and driving product improvement.
SurveyPal is a trusted tool for businesses implementing text analysis to improve support performance and quality. It delivers automatic text analytics insights allowing you to focus on your customers.
Extract meaningful insights from your qualitative support data
Perform automated text analysis in a matter of minutes
Did you like the post?
You might also like:
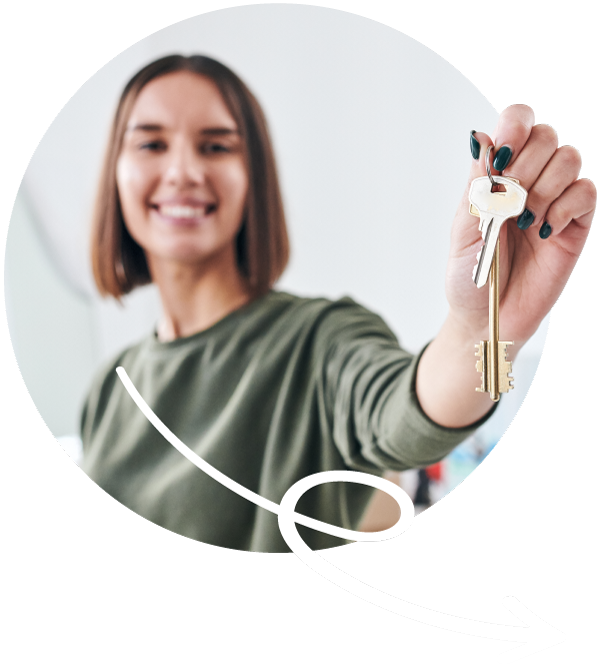
Surveypal
Everything you need to lead and improve your customer experience. Learn more at surveypal.com, or